Here’s what Google’s John Mueller says:
He seems to be implying that Google uses anchor text to help understand the context of the link and thus, it may be a “ranking factor.” That’s not a big surprise—Google’s original patent states that they use anchor text to influence rankings. (More on that later!)
Here’s another tweet from John where he reiterates the importance of anchor text:
The question is: what kind of anchor text should you use? Should you limit the use of a specific type of anchor text if you want to rank in search engines? Should you even be manipulating anchor text at all?
In this guide, we’ll reveal what we found by studying the anchor texts of backlinks to 384,614 web pages. But first, let’s make sure we understand the basics.
Anchor text refers to the clickable words used to link one web page to another.
Example: In this sentence, the blue words are the anchor text.
Say that someone decided to link to our backlink checker.
Our primary target keyword for that page is “backlink checker,” for which there are 80K global monthly searches according to Ahrefs’ Keywords Explorer.
But not every person will link to this page in the same way. Here are some of the anchor text variations they might use:
Exact Match: the anchor text is the exact keyword or phrase for which we want to rank.
Ahrefs’ Backlink Checker is one of my favorite SEO tools.
Phrase Match: the anchor text contains the keyword phrase for which we want to rank.
Ahrefs’ Backlink Checker is one of my favorite SEO tools.
Partial Match: the anchor text has all words in the query, but not as an exact phrase.
Ahrefs’ Checker de la Backlink is one of my favorite SEO tools.
Branded: the anchor text is the name of our brand:
Ahrefs’ Backlink Checker is one of my favorite SEO tools.
Naked URL: the anchor text is the raw, ‘naked’ URL (i.e., as it would appear in a browser):
Ahrefs’ Backlink Checker (https://ahrefs.com/backlink-checker/) is one of my favorite SEO tools.
Random: the anchor text is an unspecific, generic phrase which does not include our target keyword (e.g., “click here,” “this site,” “this article,” etc.)
Ahrefs’ Backlink Checker is one of my favorite SEO tools. Click here to try it for yourself!
Image links: the anchor text is the alt text of the image (according to Google).
<a href=”https://ahrefs.com/backlink-checker”>
<img src=”/backlink-checker.png” alt=”Backlink Checker”/>
</a>
For example, if we check the Anchors report in Ahrefs’ Site Explorer for our backlink checker, we can see many of the anchor text types mentioned above.
Site Explorer > Enter URL > Anchors
Now let’s take a look at how that medley of anchor texts may influence our rankings.
Google uses external anchor text to help understand what your page is about and also, for which keywords it should rank. How do we know this?
Here’s an excerpt from the original paper on which the Google algorithm is based:
Google employs a number of techniques to improve search quality including page rank, anchor text, and proximity information.
So if I linked to a page from this article with “dog biscuits” as the anchor text, that would indicate to Google that the linked page likely has something to do with dog biscuits.
If other people do the same thing, then that will increase Google’s confidence that the page in question should potentially rank for “dog biscuits.” After all, what are the chances of two or more unrelated websites linking to the same web page with the same anchor text if the page doesn’t have anything to do with dog biscuits? Pretty slim, I’d say.
Hopefully, you’re starting to see why anchor text makes sense as a ranking factor.
But of course, nothing in SEO is ever that simple.
Early Google’s (over) reliance on anchor text
Anchor text was weighted heavily in Google’s original algorithm.
In their 1998 paper, Google founders Sergey Brin and Larry Page explained:
The text of links is treated in a special way in our search engine. Most search engines associate the text of a link with the page that the link is on. In addition, we associate it with the page the link points to. This has several advantages. First, anchors often provide more accurate descriptions of web pages than the pages themselves.
Using anchor text also allowed Google to determine the topic of media formats where typical on-page signals couldn’t be used.
Second, anchors may exist for documents which cannot be indexed by a text‐based search engine, such as images, programs, and databases. This makes it possible to return web pages which have not actually been crawled.
The logic was sound, and the results were impressive, especially compared to the competition at the time—a fact that didn’t go unnoticed by the founders themselves.
While a complete user evaluation is beyond the scope of this paper, our own experience with Google has shown it to produce better results than the major commercial search engines for most searches.
But Google soon found that anchor text was VERY open to manipulation.
To rank a web page for a query, people only have to point multiple links at it with their target keyword as the anchor.
More keyword-rich anchor text links than your competitor = WIN.
This led to some amusing examples of “Google Bombing”—where SEOs would show how easy it was to game Google by pointing anchor text links at non-relevant pages and ranking them.
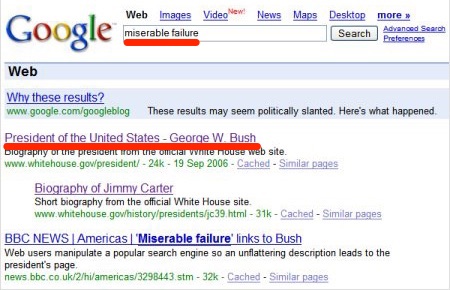
George Bush ranking #1 for the term “miserable failure” thanks to a successful “Google bomb.”
Clearly, things had to change.
Google fights back against manipulative anchor text
In April 2012, Google rolled out the first iteration of their now infamous Penguin algorithm.
Anchor text was one of Penguin’s primary targets.
Some websites that had been overly-aggressive with their exact-match anchor text links saw their rankings tank overnight. However, according to Google, it only affected 3.1% of search queries.
But things didn’t stop there…
Google continued to battle manipulative anchor text spam with subsequent Penguin updates.
Most SEOs now seem to recommend using exact-match anchor text sparingly—usually between ~1% and 5%.

Example of recommended exact-match anchor text % from another SEO blog.
So what’s the truth? Should you keep exact-match anchors to a minimum? Should you avoid exact-match anchors altogether? What about phrase-match and other types of anchors—how should you be using those?
To find out, we conducted two studies.
To study correlations between anchor text types and rankings, we looked at the top 20 search results across 19,840 keywords.
That means we analyzed 384,614 web pages in total!
All of these keywords:
- Have 2K-5K monthly searches (randomly selected!);
- Consist of 2-4 English words;
- Contain no special characters (e.g., !#@);
- Are non-numeric (i.e., keywords like phone numbers are filtered out)
Furthermore, we only selected keywords where the top 10 search results have similar URL Rating (UR) values. The aim of that was to “isolate” the anchor text variable.
Let me explain with a crude example.
Let’s say that the top 10 search results for “best protein powder” look like this:
- Exact anchor match %: 100%. UR score: 60
- Exact anchor match %: 90%. UR score: 55
- Exact anchor match %: 80%. UR score: 50
- Exact anchor match %: 70%. UR score: 45
- Exact anchor match %: 60%. UR score: 40
- Exact anchor match %: 50%. UR score: 35
- Exact anchor match %: 40%. UR score: 30
- Exact anchor match %: 30%. UR score: 25
- Exact anchor match %: 20%. UR score: 20
- Exact anchor match %: 10%. UR score: 15
You can see that the percentage of exact-match anchor text correlates with rankings. From this, we could infer that the exact-match rate affects rankings.
However, this is misleading because there’s also a correlation of UR.
So it’s likely that the number and quality of backlinks (or internal links) is also part of the reason for this correlation.
On the other hand, if the results look like this…
- Exact anchor match %: 100%. UR score: 30
- Exact anchor match %: 90%. UR score: 25
- Exact anchor match %: 80%. UR score: 32
- Exact anchor match %: 70%. UR score: 33
- Exact anchor match %: 60%. UR score: 28
- Exact anchor match %: 50%. UR score: 31
- Exact anchor match %: 40%. UR score: 31
- Exact anchor match %: 30%. UR score: 27
- Exact anchor match %: 20%. UR score: 36
- Exact anchor match %: 10%. UR score: 29
… then the likeliness of any potential correlation being caused by backlinks (or internal links) is much lower.
Make sense?
Good. Let’s take a look at the results.
Influence of exact-match anchors
First, we looked at the average and median percentage of exact-match anchored backlinks per ranking position—calculated against the total number of backlinks to the URL.
So it appears that there’s a pretty clear correlation here, right? Not so fast.
The blue line that appears to show the correlation is the average. That is a ‘biased’ representation of the points that belong to each position because extreme values can easily skew the average.
Did I lose you?
Imagine that we have the following sample of pages for position #1:
- Page 1: 0% exact-match anchors;
- Page 2: 0% exact-match anchors;
- Page 3: 0% exact-match anchors;
- Page 4: 0% exact-match anchors;
- Page 5: 100% exact-match anchors;
Average exact-match anchors for this sample = 20%.
You can see that this isn’t very representative of the entire sample—one value is skewing the average quite dramatically. That’s why we also added the median values to the graph (orange line). You can see that the median for each ranking position is zero.
Here’s what Loveme Felicilda—our data scientist—had to say about this:
I would say the average is not a good way to represent correlation. That’s why I also show the median. The fact that the median across all positions is zero means there are lots of pages with no exact-match backlinks. If our median values were to show the same “pattern” as our average, then we would have a strong correlation. So if we want to present correlations, then we should plot all the points on the graph and add a line of best fit.
That’s precisely what we did:
Now you can see that the real correlation is quite weak.
To add to that point, below is a histogram of correlations. The x-axis shows bucketed correlation values, and the y-axis shows the number of SERPs/keywords that belong to each bucket.
Generally speaking, the more bell-shaped and symmetrical the graph, the closer the correlation to the middle value (in this case, that’s zero). If it leans to the right, it’s more positively correlated. If it leans to the left, then it’s negatively correlated.
You can see that in this case, it leans slightly to the right—that indicates a weak positive correlation.
How weak? Here are the results of the Spearman correlation:
Spearman correlation (average): 0.1436
Spearman correlation (median): 0.1869
Result: there’s a relatively weak correlation between the percentage of exact-match anchored links and rankings. Both the mean and the median indicate this.
But why is this?
If Google uses anchor text as a ranking factor—or at least to understand the context of a page, as John Mueller stated—then shouldn’t there be a bigger correlation?
Not necessarily. John never said how much weight they give anchor text in their algorithm.
Furthermore, there’s a potential, somewhat unavoidable flaw in our data that I want to be transparent about. I’ll go into that shortly.
First, let’s take a look at the numbers for other anchor text types…
Influence of phrase-match anchors
To recap, phrase-match anchors are those which contain the target query.
For example, if the keyword were “SEO tool” then “best SEO tool” or “my favorite SEO tool” would both be phrase-match anchors. Let’s see how these stack up.
Two things stand out here:
- The “correlation” of the average is similar to exact-match.
- The average percentage of phrase-match anchors is slightly higher than it is for exact-match.
But again, in order to see the “real” correlation, we need to look at some different graphs:
So this time, the correlation is even lower than exact-match anchors.
Spearman correlation (average): 0.1057
Spearman correlation (median): 0.1393
Result: There’s a very weak correlation between the percentage of phrase-match anchors and rankings.
Influence of partial-match anchors
Partial-match anchors are those that contain all the words in the query but not as an exact phrase.
For example, if the keyword were “SEO tool” then “best tool for SEO” or “my favorite SEO trick is to use this tool by Ahrefs” would both be phrase-match anchors.
Let’s see how these stack up.
It looks like there’s a similar correlation once again when it comes to the average, and the median is still flatlining at zero.
Further, the average percentage of partial-match keywords is quite high compared to both exact and phrase-match percentages.
This makes sense because partial-match incorporates both exact and phrase-match keywords.
Here are the two graphs that show a better representation of the correlation:
The correlation here is almost identical to the phrase-match correlation:
Spearman correlation (average): 0.1076
Spearman correlation (median): 0.1393
Result: a very weak correlation.
Influence of random anchors
Random anchors are those which contain unspecific or generic phrases. They do not contain the target keyword (or any elements of it).
If the keyword was “SEO tool” then “click here” or “this article” would both be random anchors.
Let’s see how these stack up.
The first thing you’ll notice is that the average percentage of random anchors is super high compared to the other types of anchor text we studied. This makes sense because random anchors incorporate pretty much all other anchors besides a few very specific ones.
You’ll also notice that the average seems to indicate some correlation (albeit weak) between rankings and the percentage of random anchors.
I think this is the best example of why looking at averages is a bad idea.
Let’s take a look at some more reliable graphs to judge the true correlation.
You can see that the histogram seems to be leaning neither left nor right for this one. That means that the correlation tends towards the middle value—which is zero.
Here are the Spearman correlations:
Spearman correlation (average): 0.0161
Spearman correlation (median): 0.0130
Result: there is effectively no correlation.
That one’s hardly surprising.
If you’re familiar with the Backlinks report in Ahrefs Site Explorer, then you’ll know that we show both the anchor text of a link and the surrounding link text.
Why is that relevant?
In 2004, Google filed a patent entitled “Ranking base on reference contexts.”
Here’s an interesting excerpt from said patent:
[…] Data surrounding the link, data to the left of the link or to the right of the link, or anchor text associated with the link may be used to determine the context associated with the link.
In other words, if the actual anchor text happens to be random and unrelated to the linked page, Google may look at the surrounding link text to help understand what the page is about.
You can see how that might work with the example depicted in the screenshot above. The anchor text is random/generic, but the surrounding link text offers some context.
With that in mind, we thought it’d be interesting to study if there was any correlation between rankings and the occurrence of the keyword in the surrounding link text.
So here’s what we did:
We took the same set of keywords from study #1 but included only pages with random anchors—i.e., pages without any exact/phrase/partial/etc. anchored links.
That left us with 27,156 web pages.
To minimize bias, we further reduced our sample to 16K pages—800 in each position (1-20). This was to ensure that any correlations were based on the same number of pages for each ranking position.
Let’s take a look at what we found.
Influence of exact-match keyword in surrounding link text
Say that our target keyword is “SEO tool.”
Here’s an example of a link with the exact-match keyword in the surrounding link text:
Ahrefs’ Backlink Checker is my favorite SEO tool.
Now let’s look at the results.
You can see that there is virtually no correlation here.
Spearman correlation (average): 0.0640
Result: having the exact-match keyword in the surrounding link text appears to have no notable effect on rankings.
Influence of partial-match keyword in surrounding link text
This time we took a look at the correlation between the occurrence of all terms from the target keyword and rankings—i.e., partial match.
For example, if our keyword were “SEO tool,” then this link would fall into the bucket:
Ahrefs’ Backlink Checker is my favorite tool for SEO.
Make sense?
Here are the results:
Spearman correlation (average): 0.0205
Result: there is almost zero correlation between rankings and the occurrence of all words from the query in the surrounding link text.
Influence of 1+ words from the target query in surrounding link text
Finally, we looked at the correlation between rankings and the occurrence of at least one term from the target query in the surrounding link text.
For example, if our keyword were “SEO tool,” then all of these links would fall into this bucket:
Ahrefs’ Backlink Checker is my favorite marketing tool.
Ahrefs’ Backlink Checker is my favorite way to check SEO backlinks.
Ahrefs’ Backlink Checker is my favorite tool for SEO.
Ahrefs’ Backlink Checker is my favorite SEO tool.
You can see that this incorporates exact, phrase and partial-matches too.
Here are the results:
Interestingly, the average percentage of links with at least one word from the target query in the surrounding link text is quite high—20-25% for all ranking positions.
Still, it’s important to note that the median is zero, meaning that most of the pages we studied had no links with one or more words from the target query in the surrounding text.
As for the correlation with rankings:
Spearman correlation (average): -0.0701
Result: a slight negative correlation, but it’s so close to zero that this is effectively no correlation.
No study is perfect, and ours is no exception. Let me explain why.
Say that we wanted to know how many backlinks with exact-match anchors there are to our guide to finding email addresses.
That sounds like an easy task… until you consider the fact that the post ranks for 7K+ keywords!
So which one of those 7,205 keywords should we study as our exact-match phrase?
I know what you’re thinking: the main target keyword here is clearly “find email address,” so surely we should study the number of backlinks with that phrase as the anchor text, right?
That’s a somewhat logical assumption, but there are two issues:
Firstly, while we can easily do that for this page because we know the main target keyword, how are we supposed to do the same thing, at scale, for 384,614 web pages? We can’t, and we didn’t, because there’s no way to know for certain the main keyword that those pages are targeting.
In Ahrefs Keywords Explorer, we show the top 10 ranking pages for the target keyword in our SERP overview, plus a bunch of SEO metrics including the “Top keyword.”
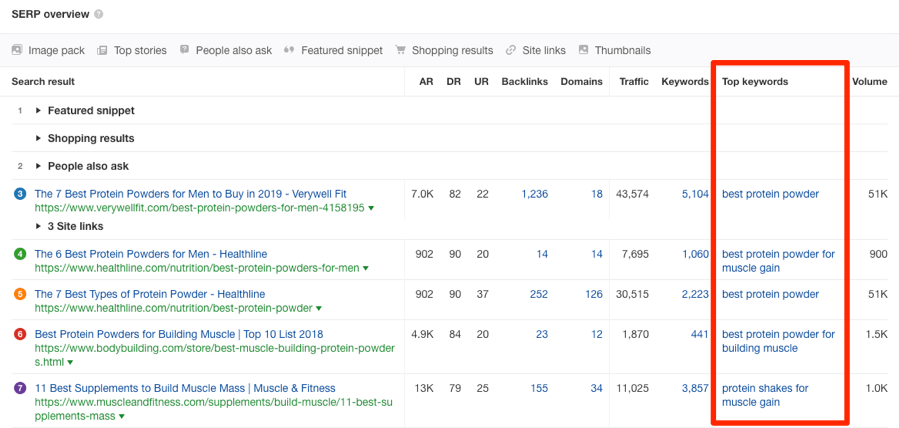
SERP overview in Ahrefs Keywords Explorer.
The “Top keyword” is the keyword that accounts for the most organic traffic to that page.
So, why not use this metric to overcome the first issue with our anchor text study?
Answer: Because the “Top keyword” only shows which keyword happens to send the most organic traffic to the page, and that isn’t always the keyword for which the author intends to rank.
Also, I think it’s fair to say that many anchored links, especially exact-match ones, are the result of “link manipulation”—i.e., SEOs building links with their target keyword as the anchor, with the intention of boosting that page’s ranking in search engines for said keyword.
Put those two things together, and you can see why using the “Top keyword” wouldn’t solve our issue.
Secondly, our sample of 384,614 webpages came from looking at the top 20 ranking pages for 19,840 keywords. However, all of those keywords matched a set of initial criteria, one of which was a monthly search volume between 2,000 and 5,000. That criterion alone surely excludes some pages’ main target keywords. In fact, that’s the case for “find email address,” which has a monthly search volume of 5,500 in the US.
Now, before you assume that the page I used to illustrate this point is an outlier and that most pages don’t rank for so many keywords, take a look at this:
We studied 3 million random search queries and found that, on average, the top 10 ranking pages also rank for between 400 and 1,300 other queries.
So, clearly, this is a large-scale happening that our study fails to take into account.
Which brings me neatly to the section you’ve probably all been waiting for…
Let’s assume that there was some magical way of knowing the primary target keyword for each page we studied, would that change anything? It’s impossible to say, but is that even the right question to ask?
I don’t believe it is, and I don’t believe that aiming to build keyword-rich anchors is a good strategy for 2019.
Here are three reasons why:
1) Topics > keywords
Here’s an interesting fact:
On average, across all posts on the Ahrefs blog, only 22% (~⅕) of traffic comes from the main target keyword.
So even if our study had no flaws, and we found that using exact-match anchors, say, 13% of the time is the secret to ranking for your target keyword (it isn’t, just to be clear), then logically, focusing on building keyword-rich links still shouldn’t be your focus.
That’s because it’s obviously an oversight to focus your efforts improving the ranking of a single keyword—which will only be responsible for sending a small percentage of that page’s total traffic.
But why is this the case anyway? Why don’t our pages—and others’ pages—see a higher percentage of total traffic coming from the primary target keyword?
Let me explain…
Google’s understanding of natural-language queries is arguably better than ever. In part, that’s thanks to the introduction of Hummingbird in 2013, which “places greater emphasis on natural language queries, considering context and meaning over individual keywords,” according to Wikipedia.
Because of this, pages that rank for their target keyword also tend to rank for a bunch of long-tail variations, which, when combined, are often responsible for the vast majority of traffic to the page.
To give one example, here’s the total US organic traffic to our guide to finding emails, via Ahrefs Site Explorer:
And here’s the organic traffic from the target keyword:
1,076/ 6,200 = ~17% of total traffic coming from the target keyword.
So, to bring this full-circle:
Exact-match anchors can only target one keyword by definition, and in 2019, ranking for one keyword is not what SEO is all about, and nor should it be your primary goal.
Recommended reading: How To Do Keyword Research for SEO — Ahrefs’ Guide
Having said that, some of you may have spotted a possible flaw in this argument. Or to be more accurate: a counter-argument.
It goes something like this:
If you could convince Google’s that your page is about x by building links with “x” as the anchor text, and Hummingbird associates x with y, and z, then doesn’t building keyword-rich links indirectly increase Google’s confidence that your page serves as a relevant result not only for x, but also y, z, and any other related queries, and thus has the potential to increase rankings and traffic across the board?
That could be true, but it’s certainly a risky and unnecessarily difficult way to achieve that outcome—especially post-Penguin.
It would be much easier to do some on-page SEO and optimize for topically-related keywords (i.e., not only x, but also y and z).
2) Risk
Building links with keyword-rich anchors is risky.
And yes, I do mean building links…
I think we all know that someone naturally linking to your page using the exact target keyword as the anchor is a rare occurrence. Which brings me to a related point:
It’s difficult to build such links without resorting to low-quality black-hat tactics like using PBNs, which is not something we advocate.
3) Weak correlations
Any potential flaws aside, the results of our study indicate that anchor text plays a rather insignificant role when it comes to ranking in 2019.
Final Thoughts
Anchor text is a complex topic. Many people in the industry continue to swear by higher-than-average exact-match anchors, whereas others—like myself—tend to think these things are better kept on the safe side.
Some people even analyze the anchor text ratios of the current top ranking pages for their target keyword and base their own anchor text ratios on the findings.
However, that’s not something we recommend for one overarching reason:
You have no control over the anchor text used with almost all legitimate white-hat link building strategies. In fact, guest blogging is the only strategy that comes to mind where you get to choose the anchor text of your links—and you should probably use branded links there, at least for author bios.
Bottom line: Your best bet for creating a natural anchor text ratio in your backlink profile—which is what Google wants to see—is simple: Don’t try to manipulate your anchor text ratios at all.